- | 9:00 am
A cyber intelligence expert explains why humans still can’t trust AI
AI systems have a significant limitation: Many of their inner workings are impenetrable, making them fundamentally unexplainable and unpredictable.
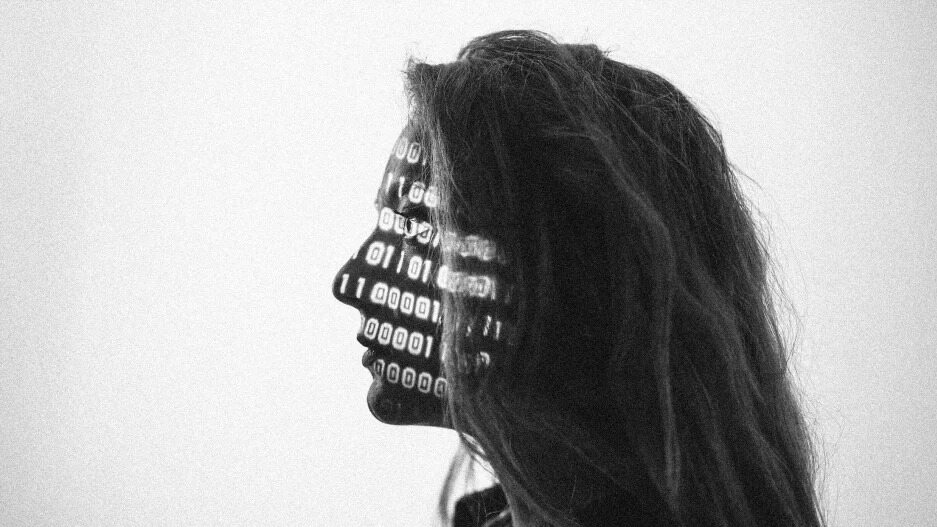
There are alien minds among us. Not the little green men of science fiction, but the alien minds that power the facial recognition in your smartphone, determine your creditworthiness, and write poetry and computer code. These alien minds are artificial intelligence systems, the ghost in the machine that you encounter daily.
But AI systems have a significant limitation: Many of their inner workings are impenetrable, making them fundamentally unexplainable and unpredictable. Furthermore, constructing AI systems that behave in ways that people expect is a significant challenge.
If you fundamentally don’t understand something as unpredictable as AI, how can you trust it?
WHY AI IS UNPREDICTABLE
Trust is grounded in predictability. It depends on your ability to anticipate the behavior of others. If you trust someone and they don’t do what you expect, then your perception of their trustworthiness diminishes.
Many AI systems are built on deep learning neural networks, which in some ways emulate the human brain. These networks contain interconnected “neurons” with variables or “parameters” that affect the strength of connections between the neurons. As a naïve network is presented with training data, it “learns” how to classify the data by adjusting these parameters. In this way, the AI system learns to classify data it hasn’t seen before. It doesn’t memorize what each data point is, but instead predicts what a data point might be.
Many of the most powerful AI systems contain trillions of parameters. Because of this, the reasons AI systems make the decisions that they do are often opaque. This is the AI explainability problem—the impenetrable black box of AI decision-making.
Consider a variation of the “Trolley Problem.” Imagine that you are a passenger in a self-driving vehicle, controlled by an AI. A small child runs into the road, and the AI must now decide: run over the child or swerve and crash, potentially injuring its passengers. This choice would be difficult for a human to make, but a human has the benefit of being able to explain their decision. Their rationalization—shaped by ethical norms, the perceptions of others and expected behavior—supports trust.
In contrast, an AI can’t rationalize its decision-making. You can’t look under the hood of the self-driving vehicle at its trillions of parameters to explain why it made the decision that it did. AI fails the predictive requirement for trust.
AI BEHAVIOR AND HUMAN EXPECTATIONS
Trust relies not only on predictability, but also on normative or ethical motivations. You typically expect people to act not only as you assume they will, but also as they should. Human values are influenced by common experience, and moral reasoning is a dynamic process, shaped by ethical standards and others’ perceptions.
Unlike humans, AI doesn’t adjust its behavior based on how it is perceived by others or by adhering to ethical norms. AI’s internal representation of the world is largely static, set by its training data. Its decision-making process is grounded in an unchanging model of the world, unfazed by the dynamic, nuanced social interactions constantly influencing human behavior. Researchers are working on programming AI to include ethics, but that’s proving challenging.
The self-driving car scenario illustrates this issue. How can you ensure that the car’s AI makes decisions that align with human expectations? For example, the car could decide that hitting the child is the optimal course of action, something most human drivers would instinctively avoid. This issue is the AI alignment problem, and it’s another source of uncertainty that erects barriers to trust.
CRITICAL SYSTEMS AND TRUSTING AI
One way to reduce uncertainty and boost trust is to ensure people are in on the decisions AI systems make. This is the approach taken by the U.S. Department of Defense, which requires that for all AI decision-making, a human must be either in the loop or on the loop. In the loop means the AI system makes a recommendation but a human is required to initiate an action. On the loop means that while an AI system can initiate an action on its own, a human monitor can interrupt or alter it.
While keeping humans involved is a great first step, I am not convinced that this will be sustainable long term. As companies and governments continue to adopt AI, the future will likely include nested AI systems, where rapid decision-making limits the opportunities for people to intervene. It is important to resolve the explainability and alignment issues before the critical point is reached where human intervention becomes impossible. At that point, there will be no option other than to trust AI.
Avoiding that threshold is especially important because AI is increasingly being integrated into critical systems, which include things such as electric grids, the internet and military systems. In critical systems, trust is paramount, and undesirable behavior could have deadly consequences. As AI integration becomes more complex, it becomes even more important to resolve issues that limit trustworthiness.
CAN PEOPLE EVER TRUST AI?
AI is alien—an intelligent system into which people have little insight. Humans are largely predictable to other humans because we share the same human experience, but this doesn’t extend to artificial intelligence, even though humans created it.
If trustworthiness has inherently predictable and normative elements, AI fundamentally lacks the qualities that would make it worthy of trust. More research in this area will hopefully shed light on this issue, ensuring that AI systems of the future are worthy of our trust.
Mark Bailey is a faculty member and chair of cyber intelligence and data science at the National Intelligence University.
This article is republished from The Conversation under a Creative Commons license. Read the original article.