- | 9:00 am
Today’s AI won’t radically transform society, but it’s already reshaping business
Machine learning veteran Eric Siegel argues in his new book that the hype around AI distracts from the very real revolution happening with companies using predictive technology.
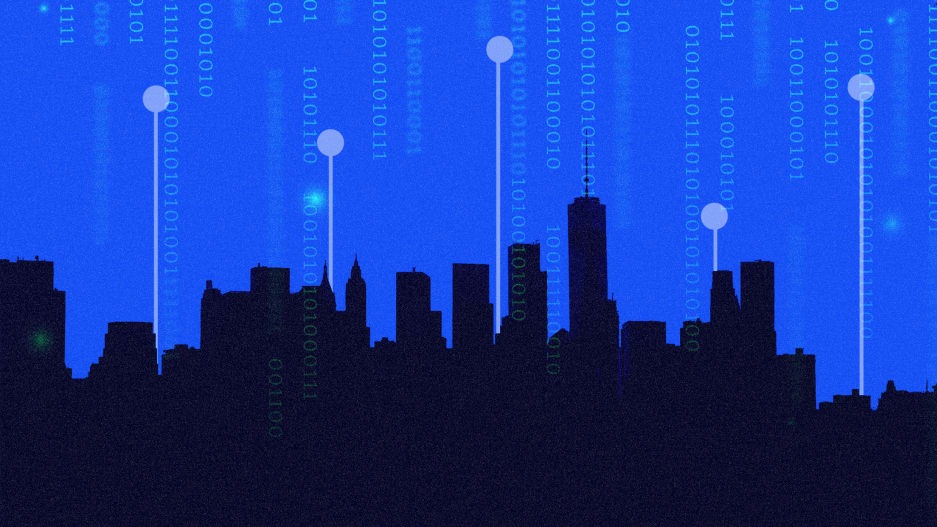
Eric Siegel had already been working in the machine learning world for more than 30 years by the time the rest of the world caught up with him. Siegel’s been a machine learning (ML) consultant to Fortune 500 companies, an author, and a former former Columbia University professor, and to him the last year or so of AI hype has gotten way out of hand.
Though the world has come to accept AI as our grand technological future, it’s often hard to distinguish from classic ML, which has, in fact, been around for decades. ML predicts which ads we see online, it keeps inboxes free of spam, and it powers facial recognition. (Siegel’s popular Machine Learning Week conference has been running since 2009.) AI, on the other hand, has lately come to refer to generative AI systems like ChatGPT, some of which are capable of performing humanlike tasks.
But Siegel thinks the term “artificial intelligence” oversells what today’s systems can do. More importantly, in his new book The AI Playbook: Mastering the Rare Art of Machine Learning Deployment, which is due out in February, Siegel makes a more radical argument: that the hype around AI distracts from its now proven ability to carry out powerful, but unsexy tasks. For example, UPS was able to cut 185 million delivery miles and save $350 million annually, in large part by building an ML system to predict package destinations for hundreds of millions of addresses. Not exactly society-shattering, but certainly impactful.
The AI Playbook is an antidote to overheated rhetoric of all-powerful AI. Whether you call it AI or ML—and yes, the terms get awfully blurry—the book helpfully lays out the key steps to deploying the technology we’re now all obsessed with. Fast Company spoke to Siegel about why so many AI projects fail to get off the ground and how to get execs and engineers on the same page. The conversation has been edited for length and clarity.
As someone who’s worked in the machine learning industry for decades, how has it been for you personally the last year watching the hype around AI since ChatGPT launched?
It’s kind of over the top, right? There’s a part of me that totally understands why the AI brand and concept has been so well adopted—and, indeed, as a child, that’s what got me into all this in the first place. There is a side of me that I try to reserve for private conversations with friends that’s frustrated with the hype and has been for a very long time. That hype just got about 10 or 20 times worse a year ago.
Why do you think the term “artificial intelligence” is so misleading now?
Everyone talks about that conference at Dartmouth in the 1950s, where they set out to sort of decide how they’re going to create AI. [Editor’s note: In 1956, leading scientists and philosophers met at the “Dartmouth Summer Research Project on Artificial Intelligence.” The conference is credited with launching AI as a discipline.] This meeting is almost always reported on and reiterated with reverence.
But, no—I mean, the problem is what they did with the branding and the concept of AI, a problem that still persists to this day. It’s mythology that you can anthropomorphize a machine in a plausible way. Now, I don’t mean that theoretically, that a machine could never be as all-capable as a human. But it’s the idea that you can program a machine to do all the things the human brain or human mind does, which is a much, much, much more unwieldy proposition than people generally take into account.
And they mistake [AI’s] progress and improvements on certain tasks—as impressive as they truly are—with progress towards human-level capability. So the attempt is to abstract the word intelligence away from humanity.
Your book focuses on how companies can use this technology in the real world. Whether you call it ML or AI, how can companies get this tech right?
By focusing on truly valuable operational improvements by way of machine learning. We see that focus on concrete value and realistic uses of today’s technology. In part, the book is an antidote to the AI hype or a solution to it.
So what the book does is to break it down into a six-step process that I call BizML, the end-to-end practice for running a machine learning project. So that not only is the number-crunching sound, but in the end, it actually deploys and generates a true return to the organization.
You write in the book: “ML is the world’s most important technology. This isn’t only because it’s so widely applicable. It’s also because it’s a novel boost that can’t be found elsewhere, a critical edge in what is becoming a final battleground of business: process optimization.” So “process optimization” sounds like the most anti-AI thing possible. In five years, what do you think the impact of AI or ML will be on the world? Process optimization seems to suggest things will mostly get a bit more efficient and seamless.
Now, the way you phrase the question kind of implies that maybe we’re only talking about incremental improvements. And I have a couple ways to address that. First of all, there’s plenty of cases where AI or ML’s impact is a lot more dramatic than incremental. If you compare a targeted marketing campaign to a mass marketing campaign that doesn’t have any particular data-driven targeting, you’re gonna see situations where the profit of the campaign increases by a factor of five. And that’s rather dramatic.
There’s also new capabilities [of AI], right? So there’s a degree to which we’re headed towards self-driving cars—even though that is going to take 30 years, not three. But it’s very important. And new capabilities like that—and many others—are only enabled by way by machine learning.
And then lastly, I’ll say that even when it’s sort of an incremental thing—like let’s say, AI or ML gives a company kind of a 1% improvement—a lot of the times that’s the last remaining way to improve. The company’s operations could be so streamlined and it’s such a large-scale established process that a 1% improvement translates into millions of dollars. We’re sort of at that stage for some kinds of operations that incremental improvement is the holy grail.
So it seems like you would be more in the camp that believes AI / ML is going to really boost productivity over the next 10 to 15 years?
Absolutely, I mean, it has in so many ways and that trajectory will continue. And that’s part of what I’m trying to do with the book. Outside of the companies that are already high-tech—the rest of the world is having trouble catching up, because they don’t have the wherewithal or the experience [in ML].
And that’s just to say, this is not just a matter of having the best core technology. There’s a business or organizational practice and discipline needed. And that’s what the book is saying: “Hey, look, this is what it takes.” You can’t just say that you’re going to buy this great tech off the shelf. The value of ML is only realized when you improve operations with it. And it’s a business practice.
In your book, you mention survey data suggesting eight of 10 ML projects fail to deploy. And I think you quoted another practitioner estimating that only one out of five ML projects ultimately succeed and provide value. Why do so many fail?
The main phenomenon is a disconnect between the business stakeholder who’s in charge of operations and the data scientists. There’s a disconnect where they failed to sort of bridge this gap.
The data scientists say, “Hey, look, I’ve got this model that predicts who’s going to cancel their subscription.” And then they’ll—the stakeholders—will say, “How good is the model?” And the data scientists can only resort typically to mumbo jumbo to answer that question because they’re literally only trained to measure predictive performance of models in technical terms rather than business metrics, like profit. Data scientists usually aren’t prepared to answer questions like “How many customers are we going to save? How much money will we save? Or how much will the bottom line improve?”
And the data scientists don’t make a practice of making that translation to stakeholders—partly because it kind of opens a can of worms. There’s sort of an implicit understanding that stakeholders are just not going to get it.
This gap is almost never bridged.
So in the end, the stakeholder has to either kind of throw up their hands because they can’t bridge this communication gap. And then they’re left with a tough decision between greenlighting the ML deployment on faith, or killing the project. And killing a project is much less risky and costly, especially today when hype really lets you sweep things under the rug.