- | 8:00 am
How ‘fake’ data can make a real difference for people of color
The CTO of Appen says AI-trained data might be the solution to tech that is still mired in racial bias, and lead to safer and more equitable applications.
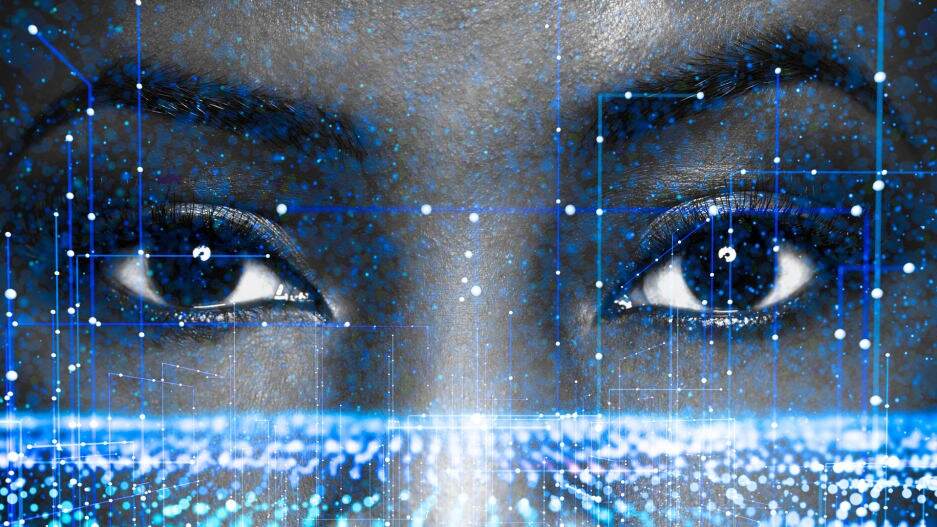
Artificial intelligence (AI) continues to demonstrate its worth, innovating operations and optimizing workloads for organizations across all industries. As more industries look to harness the power of AI, we must be extra sensitive to the data we are using to train this technology. If we aren’t, we risk backsliding against all the progress society has made in recent times in relation to intrinsic bias against Black, Indigenous, and People of Color (BIPOC).
THE RISE OF SYNTHETIC DATA
Businesses are using AI to venture into previously unexplored territory. Human-in-the-loop data training can take you a long way, but what about the cases in which we have no previous data? How can we teach an AI model to do something we don’t yet have the tools or data to do ourselves?
Originally, developers had to obtain training data that covered every possible scenario to accurately train successful AI models. If a scenario had not occurred or been captured before, there was no data, which left a huge gap in the machine’s ability to understand that specific scenario.
There are real-world scenarios that happen but are not often documented enough to have the abundance of data needed to be able to train a machine to recognize it. For example, we don’t have enough of the data needed to train an alarm system to recognize a home intruder. Another example is training an autonomous vehicle to recognize a child running out in front of the car. Although extreme, these are real-life scenarios that we can’t just train a machine to recognize and react to with human-in-the-loop data alone.
However, where there’s a will, there’s a way—and the way points down the path of synthetic data.
WHAT IS SYNTHETIC DATA?
Synthetic data is created by software as opposed to data humans captured from real-world scenarios. It enables computer programs to fill in the gaps in use cases by orchestrating rare instances and specific real-world scenarios that typical human-collected data simply cannot manifest. These are called edge cases. This also allows more freedom and flexibility when it comes to training more sophisticated AI applications.
Edge cases are the extreme, nightmare scenarios that the AI might not be prepared to handle. For example, catastrophes or crimes are both scenarios in which it is difficult to collect data. Although these can be simulated risk-free, synthetic data should be used in combination with as much real-world data as possible to close the gaps and ensure holistic, inclusive data sets for all possible scenarios.
By 2024, 60% of all AI data is expected to be synthetic data. Although the idea of synthetically generated data has been around for quite some time, its recent growth can be attributed in large part to the autonomous vehicles industry. However, it can be applied in nearly any program-leveraging computer vision, such as drones, security cameras, and various consumer electronics.
NO HUMAN MEANS NO HUMAN BIAS
Synthetic data allows companies to break away from traditional AI data limitations. When used in conjunction with human-collected data, synthetic data can offer substantial benefits to businesses, including reduced cost of data and labor, increased speed of data collection, access to edge cases, and more-inclusive, less-biased datasets.
Just as bias is an ever-standing presence in society, it holds space in AI datasets as well. Because these datasets are curated by humans, they often show the same biases as the people who create them. No, these aren’t huge, obvious biases, but they are enough to skew applications on the basis of sex and race. For example, self-driving cars are more likely to recognize white pedestrians versus Black, which can result in major safety issues.
What sets synthetic data apart is that it’s not created by humans. It’s data created for AI by software. And, although it can still inherit bias from the original set, this means it carries much less bias, if any at all.
For the dataset to be truly inclusive, it needs to cover every possible scenario and person who may use it. For example, facial recognition for your cell phone needs to be able to work for everyone, so it needs to be trained to identify skin color, hair color, hair type, different facial features, accessories like glasses or sunglasses, and more. All these variables need to be added into the training dataset to ensure inclusivity. More specifically, if we know that we don’t have data on people who wear glasses, then we can create that data artificially to ensure the models work for those wearing glasses.
Additionally, an autonomous vehicle needs to be trained on all road situations, including different types of roads, different street sign languages, different extreme experiences, and whatever else is thrown its way. While real-world data are actively being collected to train these models, there are often scenarios that are unpredictable or infrequent that the model needs to be able to recognize in order to keep everyone involved safe. Let’s say a ladder falls off the trunk in front of the vehicle, the vehicle needs to identify the object and move around it. These scenarios don’t happen enough in the real world for us to have enough data to properly train a model, but they can be created artificially through the use of synthetic data.
With synthetic data rising in popularity, the future is bright for AI. As more and more companies adopt the concept to supplement human-collected datasets, we can expect much more inclusive and representative datasets that will lead to safer and more equitable applications for all genders and races.