- | 8:00 am
How to harness generative AI for designing great customer experiences
To uncover the innovation potential of generative AI, and at the same time keep these systems in check, we need a multidisciplinary approach that combines product design with AI, machine learning, and other data-driven methods.
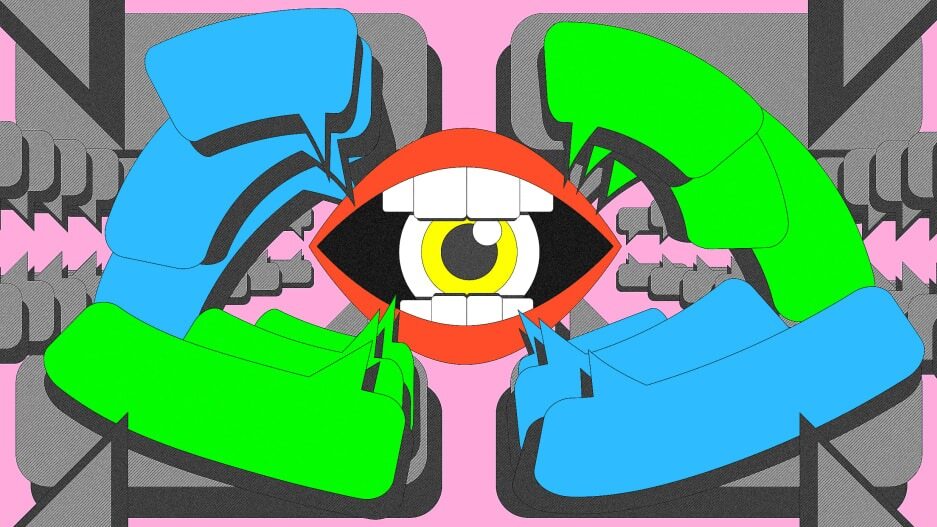
Generative AI technologies, such as large language models (LLMs) and their applications are now going mainstream: OpenAI and ChatGPT are leading the way, Meta and Alphabet also joining the race with multiple other players following suit.
Different use and business cases are explored with enthusiasm in B2C and B2B sectors from smarter search to chat-like shopping experiences, and from journalistic news content creation and personalized marketing to AI-augmented tooling for wealth management, collaboration, and education.
At the same time, this new wave of disruptive AI-driven innovation has also raised concerns varying from the upcoming AI apocalypse to more thoughtful considerations on how the effects of baked-in biases, malicious uses, and data security could cause wide scale harm to individuals, businesses, and society.
One thing has become apparent: This fast development will require new thinking and approaches for product design. For example, the way we explore and discover new solution spaces, define experiences, and develop customer experience requirements, is changing rapidly.
GENERATIVE MODELS GO BEYOND CHATBOTS
Why do generative AI technologies such as LLMs matter? To get the full picture from a product design point of view, we need to first understand their nature a bit deeper.
An LLM is a neural network-based general purpose AI that can interpret and generate natural language text. These models are predicting what word will follow the other (or in generative imagining what pixel makes sense next to another). In order to make the prediction efficiently in different contexts, these generative models have been fed and trained with huge amounts of content data such as online text materials.
However, the applications and potential uses of generative language models go beyond interpreting and generating text. Generative models have emergent abilities that can be discovered when the models are applied to new use cases and tasks. Notably, at their core LLMs are continuously learning and updating information infrastructures that can be used for dynamic information processing, manipulation, management and retrieval.
The end-game of LLM-powered experiences is not the chatbot experience that you see today popping up like mushrooms here and there. Generative models can serve as platforms to power a great variety of dynamic experiences combining different types of interactive elements and content from text and images to links, videos, visualizations, and beyond. Human-centric product design plays a key role in harnessing these powerful technologies in an impactful and sustainable way.
THE UNCONTROLLABLE SUPERPOWERS OF GENERATIVE AI
Generative AI technologies can give you superpowers for creating different kinds of content and customer experiences. However, these powers are not easy to control. Predicting or controlling the output and behavior of generative models is not straightforward for three main reasons.
These infrastructures and their inner workings are part incomprehensible and inaccessible black boxes. both for the developers and users of the existing generative model applications and services. For example, the users of the ChatGPT can’t directly affect the exact dynamics or workings of the model itself.
In generative models the information or its structure isn’t stable or static, but very dynamic. Basically, all the information within a generative language model can be manipulated and altered at a very granular level. At the same time, as the model itself is iterated and changed, its behavior and thus for example, its responses to similar input can alter. LLMs don’t provide one static source (or even one presentation) of baseline truth, but pieces of information and their relationships can be changed, combined, and mixed infinitely.
Due to their dynamic nature, generative models such as ChatGPT, Bard, or Character AI can make things up. When predicting the next piece of content in the given context, LLMs can come up with completely new combinations of words and sentences thus creating things that have nothing to do with our shared reality. Indeed, generative models are making up people, art works, job histories, companies, fashion products, and whatnot. These imaginary outputs are often called hallucinations. For some use cases and experiences this inventive power of generative models is great, for some it can be disastrous.
As such, there isn’t one consistent or right way to control or guardrail generative AI. Different companies from Microsoft to Nvidia have come up with guidelines to manage and supervise generative models. However, any of these existing frameworks can’t guarantee a fully predictable control over dynamic and creative powers of LLMs.
Given this, the most pivotal thing for product design is to find a balance between inventive powers and control to create inclusive, consistent, and meaningful customer experiences that benefit the business.
TAKING CONTROL OF SUPERPOWERS
The way for finding the balance needs to be baked into the multidisciplinary process of designing and developing LLM-powered products and experiences. Product design needs to:
- Help the organization to evaluate which customer problems and business cases can benefit from solutions that are powered by generative AI.
- Turn the generative AI black box into an aquarium by understanding deeply the interactions of customers and machine agents as well as the related data flows.
- Guide the customer by keeping the AI-powered experience human-centric, safe, and secure.
TO LLM, OR NOT TO LLM
Generative models like LLMs aren’t a silver bullet that changes things for the better overnight. You need to consciously explore, recognize, and assess what kind of customer problems and use cases would concretely, and safely, benefit from leveraging generative AI.
Get ready to progress iteratively in finding out the most fitting use cases and experiences, and then iterating on them in a pragmatic customer-centric manner.
Explore the solution space and its opportunities with an open mind, and think beyond chat bots. Map the risks and think about guardrails early on.
- What kind of biases might creep in in your particular use case and experience?
- What is the best way for making data usage transparent to customers?
- What kind of safety mechanism can you bring in the experience itself to keep the customer in control and the inventive powers of the AI at bay?
Discuss and work closely on potential solutions with your engineering and applied science colleagues to understand different risk scenarios and technological feasibility and to recognize potential low-hanging fruits.
In this phase, product design, data science, engineering, and business should work hand in hand to ensure that things are driven by customer and business first, not allowing the technology itself to take the driver’s seat.
After the preliminary use cases and preferred customer experience scenarios have been defined, the design and development work should happen in parallel to enable continuous learning and iteration.
UNBOXING THE BLACK BOX
Prompt engineering is one of today’s hot topics. In order to develop engaging customer experiences powered by generative AI, product designers need to dive deep into this emerging art.
The first thing you learn is that there is no one specific way of getting your prompting right for your use case and experience. And second, the only constant is change. For example, when the version of LLM model version of your choosing changes, your previously fine-tuned prompts might not be working anymore as expected.
From a product design perspective, prompting needs to be thought of in strategic and tactical terms.
First, you need to concretely figure out what kind of preliminary prompts and rules, as well as additional information, systems, and data sets are needed to support your specific customer experience and business case. Prompting is (almost always) just one part of the equation.
Second, you need to think through a framework that allows you to systematically design, develop and evaluate customer experience and prompting, and to debug them, too.
Prompting happens at two levels. One is a level that is visible to the customer, and another is a level in which the machine agent interprets the customer input and then potentially uses that interpretation to call other systems or leverage other data sets.
For example, you’re designing a wealth management assistant. At the first level of prompting, you need to think about what are the ways you allow your customers to interact with the assistant, and what are the potential topics the assistant can cover for them. You can allow the customer to ask the wealth management assistant about the latest hit music, but the assistant might answer that they are not equipped to give pop music tips based on how you’ve restricted the topics it can cover.
In the second level of prompting, the customer, for example, voices a question to get investment advice regarding a specific sector of information technology. You’ve prompted the assistant to be able to give advice on this specific topic in a specific tone of voice using a certain content presentation formula combining natural language and data-based infographics.
From a product design perspective it is crucial for the system to take the customer’s input and interpret it correctly in order to formulate a concise and comprehensible answer consisting of natural language and graphics. The machine agent needs to take the customer input and be able to call the right additional systems under the hood, e.g., a database that is used for creating the infographic. Relevant answers to customer’s questions can’t be surfaced should the machine agent interpret the customer input incorrectly, and for example omitting the call to the database that allows it to produce the infographic.
Product design needs to be aware of these key information flows and their dynamics in order to create more visibility for controlling the experience. For driving the design and development, you should thus create a two-layered framework that allows you assess the quality of the interactions between your customer and the LLM-powered agent, and to evaluate the interpretation of customer input that is used to connect the machine agent to the right background systems.
For effective prompting and its quality evaluation, set up your team for fast learning and pragmatic iteration. Prepare to design, develop, test, and iterate in unison. Edge cases, malfunctions, and anomalies will surface, and some of them will only be spotted by trying things out concretely. For this very reason, be prepared to get the first version of the experience in front of the selected group of real customers as soon as possible to surface error cases and customer experience pain points that allow you to iterate the solution pragmatically.
This doesn’t mean that product designers should turn into engineers or applied scientists. But again, product design should work closely with engineering and data science in developing suitable and effective prompting tactics and their end-to-end evaluation methods to ensure that the customer experience develops in the right direction. This might require setting up automated and human-in-the-loop customer experience testing frameworks that help to spot potential regression of the experience in some areas, when there might be improvement in others.
GUIDE THE CUSTOMER TO CREATE INCLUSIVE EXPERIENCES
Generative models can be used to power completely new kinds of customer experiences that introduce new ways for your customers to interact with your product and content. Managing customer expectations and guiding them in a comprehensible manner becomes essential to ensure that the new experience really helps customers in their tasks. Similarly, it is pivotal to recognize the most important customer touch points that should, for example, inform the user interface and interaction choices, messaging, and feedback loops that are in sync with prompting.
When thinking of a user interface and interaction solutions, hold the customer’s hand and let them learn at their own pace. Pay attention to how the customer gets started and the experience itself sets them up for success. Design shortcuts and safety levers that for example allow customers to escape dead-end conversations, or make sure the hallucinations of the machine agent don’t get in the way of your customer’s interests, intentions and needs.
When creating real-life customer experiences, reliability, safety, and security considerations should always be prioritized. Water-proof guard-railing and related customer-facing solutions emerge from a deep collaboration with experts that are familiar on data bias and accessibility topics.
Product design is starting to go way beyond its more traditional realm of user interface, user experience, and information architecture design. To uncover the innovation potential of generative AI, and at the same time keep these systems in check, we need a multidisciplinary approach that combines product design with AI, machine learning, and other data-driven methods.
We need to rethink product design processes to be able to create and develop truly dynamic experiences that are powered by continuously developing dynamic machine agents and various data sources.
In the longer term, we might need to reassess some of the core skills needed by product designers, as well as the structure of design organizations themselves.
Human-centric product design is crucial for making sustainable future-proof progress when leveraging generative AI to create meaningful experiences that drive your business. At the same time, it will empower the discovery of new and unknown use and business cases for generative AI models.