- | 9:00 am
80% of the information we generate becomes ‘dark data.’ This is how to bring it to light
If numeric data is only 20% of the total information available, then the opportunity to understand and utilize dark data at scale is transformational.
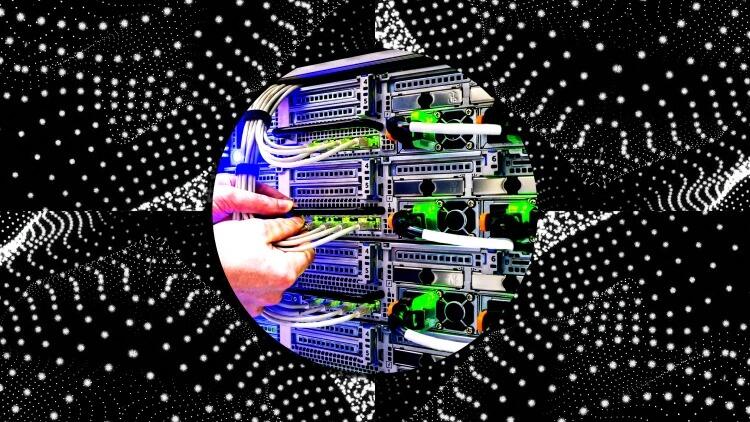
According to NASA, “matter” is any substance that has mass and occupies space. But there’s more to the universe than the matter we can see. Dark matter and dark energy are mysterious substances that affect and shape the cosmos, and scientists are still trying to figure them out.
What if we were to look at the amount of data created over the last two decades or more in the same way? If dark matter makes up 85% of the matter in the universe, in the earthly world of business intelligence and analytics, only about 20% of information is numeric and easily studied using statistical techniques. This means the other 80% is largely invisible, like dark matter, silently influencing many outcomes in business and the larger world without being subject to scientific, objective, scaled study.
Now, with the capabilities of generative AI (GenAI), and specifically large language models (LLMs), scientists can examine this unstructured, dark data, in new and exciting ways, leading to vast modern analytical capabilities that can unlock new meaning in all the world’s information. For leaders, this capability heralds a sea change and presents early AI adopters with a rare chance for true competitive advantage.
Where the dark data lives now
The hunt to civilize and harness the insights contained in dark data is well underway. In the modern digital world, a continual barrage of text data is constantly created through news and social posts. But this dark data can’t be processed at scale with traditional means.
A recent study by researchers and academia in the legal domain hypothesized that evidence of legal violations could be found hidden in most information. Various LLM and other AI approaches were used to dissect samples of the data, validating the usefulness of these tools to identify violations. Interestingly, the researchers showed that evidence of legal violations could be found using AI, and they could even associate those violations with specific victims.
Other researchers have shown that LLMs can be used to code qualitative data. Coding involves assigning a designation to text and is historically done by human raters. This takes substantial time and often involves sampling the data rather than coding all of it (not to mention being excruciatingly boring and difficult to carry out at high accuracy levels).
Once the data is coded, it can be further subjected to statistical analysis. Similarly, it has been shown that ChatGPT can be used to cheaply and efficiently code tweets with results superior to human coders. These researchers calculated that it costs $0.003 per annotation, which is about twenty times cheaper than using human coders through a mechanical turk-type process.
Turning to healthcare, consider that medical science progresses through careful analysis of highly specific numeric datasets, yet there is a tremendous cache of information to be found in images, physician notes, test results, and scientific study descriptions.
For example, researchers note there are many promising applications of LLMs, including analyzing medical studies at scale. While there are ethical considerations here (e.g., ensuring that a poorly trained AI doesn’t recommend the wrong treatment), there is also great potential to advance medical care by better utilizing patient and scientific data for things like early detection of conditions or predicting reactions to specific medication based on an individual’s unique profile.
Tapping the business opportunity
Many companies fail to adequately analyze the available numeric data, and bad data itself has been estimated to cause losses of $3.1 trillion per year to the U.S. economy alone. If numeric data is only 20% of the total information available, then the opportunity to understand and utilize dark data at scale is transformational. While GenAI can help you to summarize long documents, the real benefit of LLMs is understanding all information and using this insight to inform business decisions.
Consider customer and employer survey data, and all the open-ended comments that are largely unreviewed, or the many other types of unstructured information that are languishing in databases such as product reviews, customer feedback, job candidate profile data and resumes, expert financial analyses, corporate policies, technical manuals, legal contracts and opinions, and on and on. This dark data can now be quantified and studied, and not just once as a typical manual analysis or audit would do, but in a continual, scaled manner.
Finnish researchers recently described their attempt to determine the value of using LLMs in qualitative data analysis. In their multi-agent approach, they broke down AI tasks into several discrete steps including thematic, content, narrative, and discourse analysis, plus a step that even creates theories from the analysis. After implementing their approach with a variety of datasets, the researchers found that practitioner experts rated their automated results very highly. While this and other approaches are quite new, there is tremendous potential to leverage LLMs to make sense of unstructured datasets.
Generation vs. intelligence
Most of the tech world is stuck on the generative component of GenAI: fun images of fanciful ideas, summaries of long documents, ideas for activities at your nephew’s fifth birthday party, etc. But while entertaining and certainly labor-saving for individuals, corporate utilization, despite all the hype, is surprisingly low, and many companies creating AI tools have yet to successfully monetize their investments.
The key to understanding new efforts to civilize dark data is not the generative aspect of LLMs, but their ability to intelligently understand human commands and carry out instructions. Using a retrieval augmented generative (RAG) approach, a user can feed documents into an LLM, and then ask the LLM questions about that information or even to evaluate that information in a specific way.
Let’s say you have a thousand-page contract to review and you need to evaluate it on various compliance standards required by your employer. You can do this the old-fashioned way, which is an excruciatingly slow process prone to errors, or you can feed it into a RAG system and score it on your organization’s standards. To be clear, you have to write some code to do this and tweak it to ensure it works properly, but once done, you can use it continuously.
Up until now, AI and data analytics have worked best using structured and organized numerical data, and while there are many legacy techniques for exploring unstructured data such as sentiment analysis, topic modeling, and keyword extraction, LLMs are uniquely capable of parsing and manipulating non-numeric data.
The key aspect of LLMs that makes them so good at processing text, in particular, is that they understand and can carry out human commands to a degree never before possible. Users can instruct them to analyze a corpus of text, looking for answers to questions or specific information, and can further ask them to rate the returned findings on an anchored rating scale. The AI thus converts qualitative data, which is not subject to easy statistical analysis, to meaningful quantitative data that can be combined with native numerical data and crunched using common statistical tools.
Physicists might not be close to understanding dark matter, but businesses and researchers can now make real inroads to civilize dark data.