- | 9:00 am
AI washing is real. This is how to see through deceptive claims
Similar to greenwashing—false marketing around a product or practice’s environmental benefits—AI washing has solutions that aren’t trustworthy to capitalize on the imperative need for adoption.
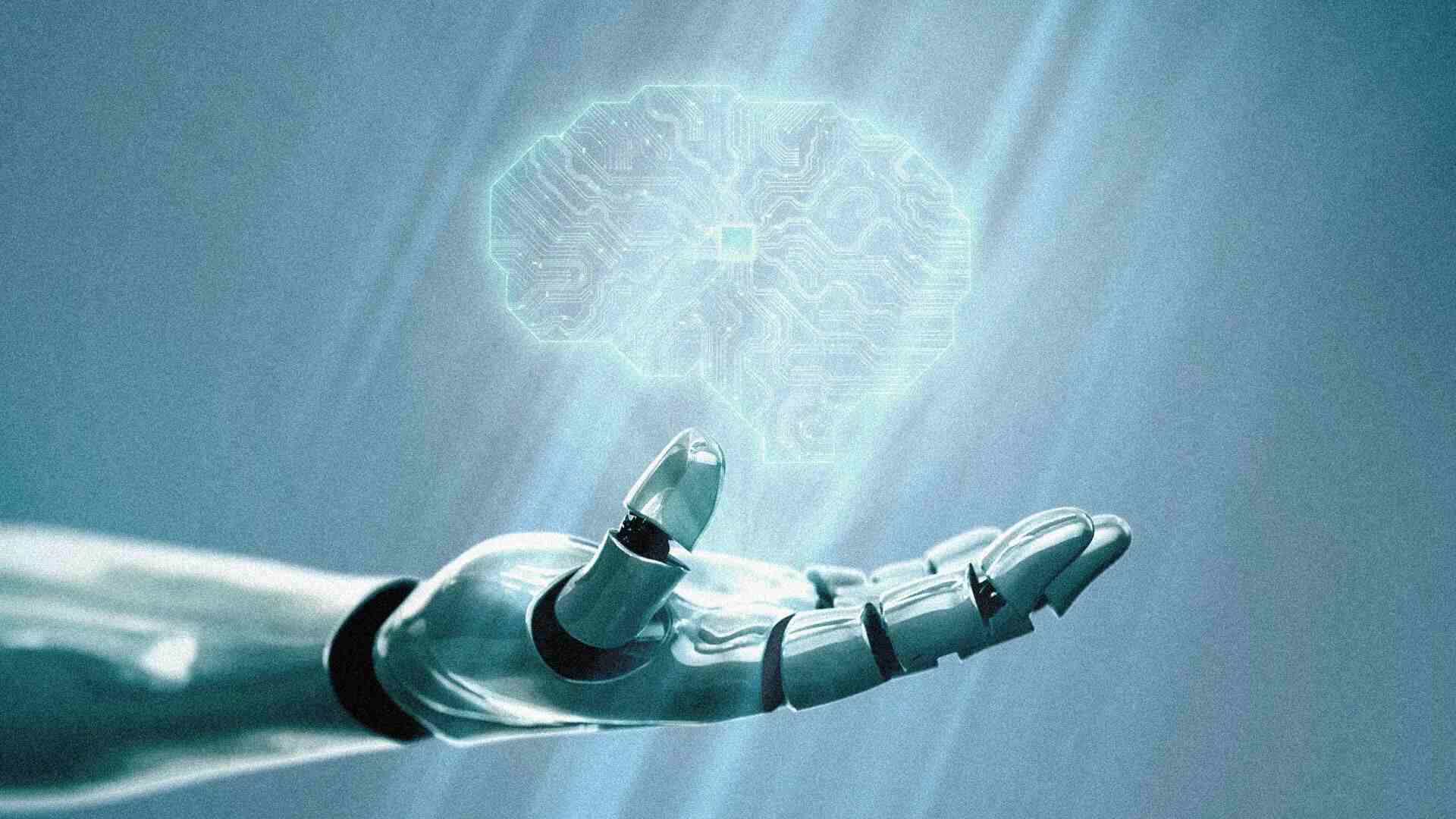
Effective use of AI technologies is this decade’s golden opportunity for companies, large and small. But as every software vendor claims to be “powered by AI,” “AI-ready,” or simply just “AI” – how does one separate potentially game-changing AI innovations from AI fiction? We call the seemingly instant AI-ification of everything “AI washing,” and business leaders need to be aware of this growing trend as they consider which tools to bring into their organizations.
With nearly every industry tapping into AI to boost efficiency and productivity, companies and their consumers are exposed to the rise of AI wannabes touting false capabilities with large language models and information retrieval tools. SEC chair Gary Gensler warned against the practice of AI washing at the end of last year, making it clear that securities laws require company disclosures to be “full, fair, and truthful.” Similar to greenwashing—false marketing around a product or practice’s environmental benefits—the more recent trend of AI washing is capitalizing on the imperative need for adoption.
A January 2024 survey conducted by Glean and research partner ISG, for example, found that nearly 80% of CIOs and IT leaders across companies in the Americas and Europe believe generative AI will be crucial to their organization’s productivity. But as companies develop and implement their AI strategies, what should both leaders and employees, who are eager to start using these tools in their day-to-day work, know about the potential of AI-washing?
WHY DOES AI WASHING HAPPEN?
The emergence and explosive growth of generative AI rapidly transformed the way people think about workplace productivity and output. The generative AI market’s overall value is poised to reach $1.3 trillion by 2032, up from $128 billion in 2024, according to Bloomberg Intelligence. The potential gains to global GDP are even greater as generative AI is projected to fuel an increase of about $7 trillion over 10 years, per Goldman Sachs.
Amid this transformational shift, investors and entrepreneurs are eager to build new products and offerings using this technology. While many companies begin with the best intentions and a grand vision of what’s possible, they can’t always turn their vision into reality.
In this crowded landscape, expectations are sky-high, and showing leadership in the market early is crucial to businesses finding success. Some entrepreneurs may try to generate and capture interest in a concept, and then validate and assess demand in the market before building the product itself—essentially finding product-market fit before they have a product.
Even for companies working on AI solutions for a while, building powerful deep learning models is a complex effort that takes significant time and resources. Creating solutions that deliver trustworthy results is a Herculean task.
BUILDING TRULY TRUSTWORTHY AI
One of the biggest barriers to greater adoption of AI is a general lack of trust in accuracy. Receiving accurate output from generative AI tools is no small engineering feat, and not all solutions that brand themselves as enterprise-ready generative AI tools are capable of delivering the accuracy needed at work.
Leading businesses—including Alphabet, Amazon, and Nvidia—have committed to responsible use of AI bolstered by transparency and public reporting of AI models and capabilities. Many small and midsize AI companies start with good intentions based on the art of the possible but can’t deliver due to the specialized skills and resources required.
Building generative AI tools that are robust and trustworthy enough to be used in the enterprise can be challenging for many reasons. In particular, large language models (LLMs) are only reasoning engines; they lack enterprise-specific knowledge. Using LLMs with enterprise data requires first retrieving the most relevant, up-to-date information from an enterprise corpus, and then feeding that data into the reasoning engine to generate accurate and useful output—an industry best practice known as “retrieval augmented generation, or RAG.
Creating and refining these systems involves training models that combine dozens of different ranking signals to determine relevance, and requires significant expertise and infrastructure. Other challenges include managing security permissions, data freshness, and the explainability of sourcing so AI answers can be understood and trusted. Developing this demands tremendous financial and operational resources, and can take some enterprises years to accomplish.
When evaluating AI tools to use at work, it’s critical to remember that not all AI tools are created equal. Look for solutions with transparent AI policies and guidelines and for companies working at this for several years. As much as you can, experiment with these tools to compare which deliver more accurate results.
WHAT TO LOOK FOR IN AI TOOLS
Beyond evaluating the accuracy of the products you’re considering, it’s also essential to evaluate how well those products will integrate with existing tools and workflows, as well as how usable they’ll be for nontechnical employees across your organization.
Many AI companies tout themselves as seamless and easy to use and present a multitude of interesting use cases like synthesizing customer feedback or summarizing meeting notes. While the products may show well in shiny demos, it’s worth investigating whether or not they’re truly intuitive and how quickly they can be adopted by teams of varying technical skill.
Additionally, it’s important to understand the amount of setup or implementation work required to use the product as intended. Some of the more unique or interesting use cases may need significant work by engineering teams over weeks or months before the tool can be used as envisioned.
Generative AI is one of the biggest transformations in technology so far. It’s not surprising that companies are clamoring to attach themselves to this technology. Responsible leaders and consumers of generative AI need to be critical thinkers and purchasers in order to avoid falling victim to AI washing.