- | 8:00 am
DeepMind’s new AlphaFold 3 expands to DNA, RNA modeling
By predicting how proteins interact with RNA and DNA, AlphaFold 3 could dramatically accelerate discovery of new drugs, better antibiotics, more effective vaccines.
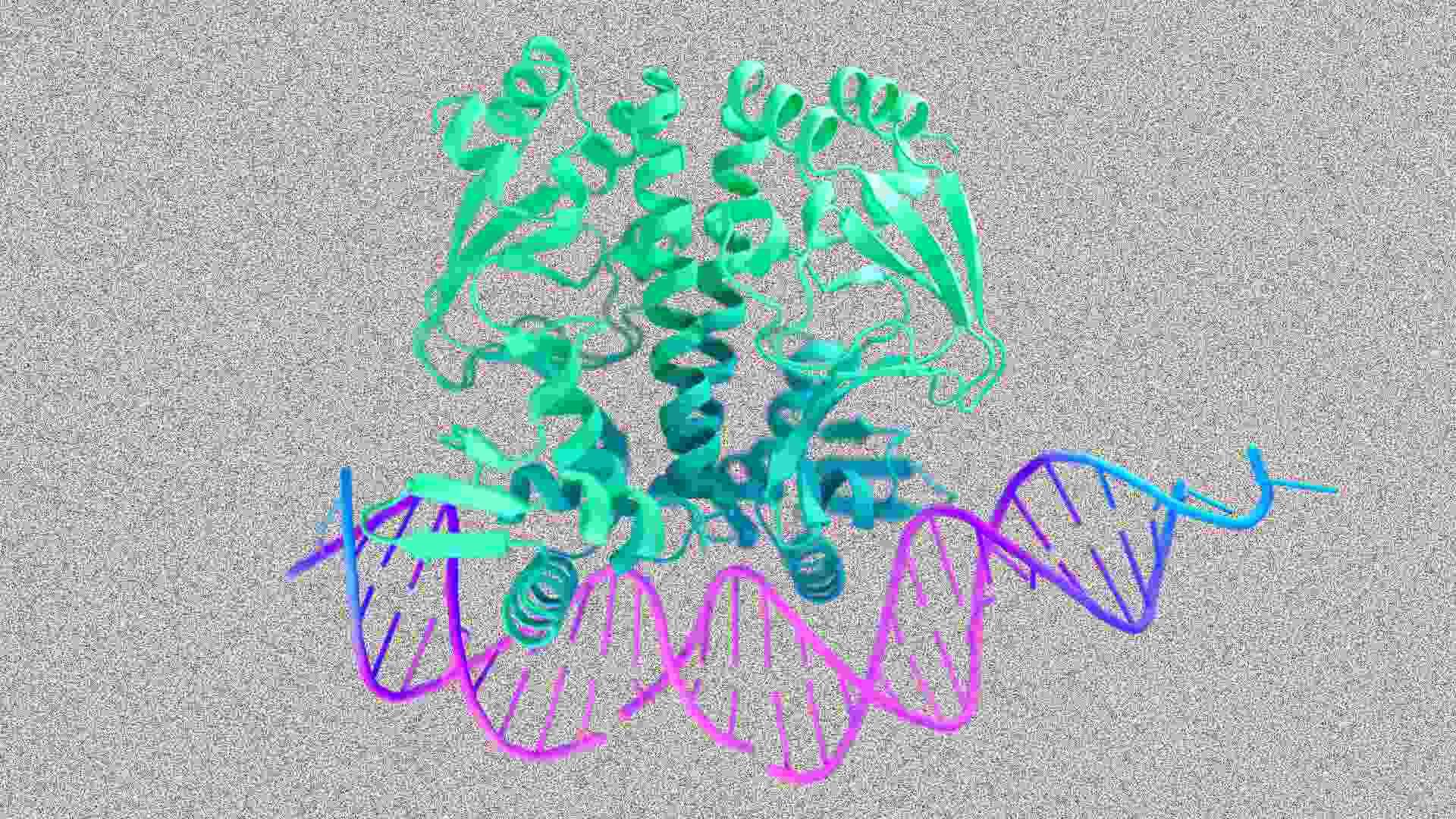
Google DeepMind has expanded its AlphaFold AI system to not only predict the structure of proteins, but also to model how proteins interact with other cell structures, including DNA, RNA, and small molecules that are often used in drugs. The new system, called AlphaFold 3, can model the ways in which proteins “read” our DNA and then carry out the instructions in the body.
AlphaFold 3, which DeepMind developed with the London-based drug developer Isomorphic Labs, provides drug researchers with a more powerful tool to model how new drug compounds might react with certain receptor sites in the body, which could accelerate the exploratory phase of drug development. Traditionally this work has been done experimentally, in a lab.
During a conference call with journalists Tuesday, DeepMind CEO Demis Hassabis described how drug researchers could use AlphaFold 3. “You imagine that AlphaFold gives you the structure of a protein you’re interested in, in a particular disease, lets say, and with these new capabilities we can now design a compound or ligand (a “chemical messenger“) that will bind to a specific place . . . on the surface of the protein, once you understand the structure of it, and we can predict how strong the binding affinity will be,” Hassabis said. “It’s a critical step if you want to design drugs.”
“This opens up what I call rational structure-based drug design,” Isomorphic chief AI scientist Max Jaderberg said on a conference call with journalists Tuesday, “the ability of our scientists to design potential new drug molecules and, with AlphaFold 3, see those results in a computer—in silica, as we say—to help the scientists reason about what interactions to make and how to advance those designs to create a good drug.”
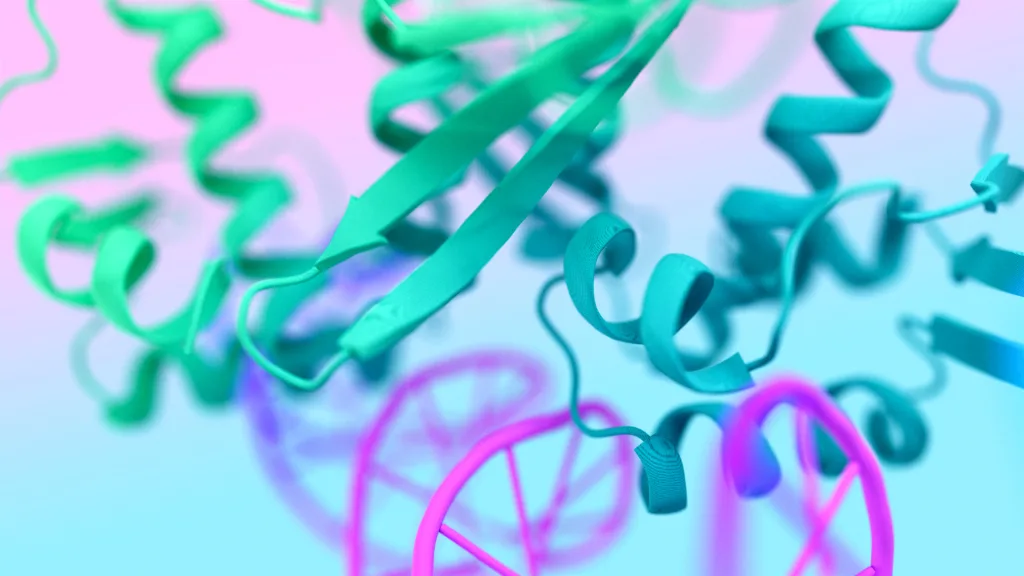
AlphaFold 3 can’t get researchers all the way to a new drug. Other AI models must be used to predict things like toxicity and interactions with other drugs. And all of a new drug’s expected interactions in the body must be proven in wet lab experiments, then in human clinical trials. But the tool can provide researchers intuition about where new R&D work should be pointed.
The tool could also be used to design food molecules that are, for example, more resistant to spoilage, or to design new, more effective, antibiotics. It could be used in the early development of new vaccines.
Instead of open-sourcing the new model, DeepMind is offering a cloud-based “AlphaFold Server” where academic researchers can access AlphaFold 3 and “generate biological structures,” the company says. This could be very useful for scientists who lack experience in bioinformatics (or have no interest in it), or lack access to the compute power needed to run the model. Hassabis said DeepMind is using the model in its drug development work with Isomorphic, which in turn is working with Novartis and Eli Lilly.
“What’s really exciting is that we’ve seen enormous advances in accuracy over other tools and even AlphaFold 2 in the different types of predictions we make,” said AlphaFold team lead John Jumper. He said that for the interactions of proteins with small (drug-like) molecules AlphaFold 3 showed 76% accuracy in a benchmark test (versus 52% for the next best tool). For predicting how proteins bind with DNA AlphaFold 3 was 65% accurate (versus 28% for the current state of the art).
AlphaFold 3 is a big leap forward for bioinformatics to be sure, but it still represents one of the first steps in a long journey toward building AI that’s capable of modeling, with high accuracy, the vast universe of possible interactions of biological structures and molecules in nature, as Hassabis acknowledges. And DeepMind may face a real challenge in pushing AlphaFold further down that road. Why? The same reason large language models could hit a brick wall–a dearth of reliable training data.
“It’s exciting that they’re able to make these types of advances really only by advancing the technology side of the equation,” says Ginkgo Bioworks head of AI Anna Marie Wagner. Wagner explains that making big leaps forward modeling biological structures and behaviors requires three main components: computing power, technology (i.e. software), and data. AlphaFold is a powerful model with Google-size compute power behind it, but, Wagner points out, it relies on publicly available laboratory test data sets for its training.
“We need to generate large, diverse data sets that are representative of more diversity that this model could train on,” Wagner says. “What I get really excited about is when you start combining these advances with having the type of multimodal data that allows these types of models to learn even faster–that’s where I think we start getting the real magic.”