- | 4:00 pm
Is Perplexity AI showing us the future of search?
Perplexity hopes to carve out a slice of the massive web search market that Google has so thoroughly dominated for 30 years.
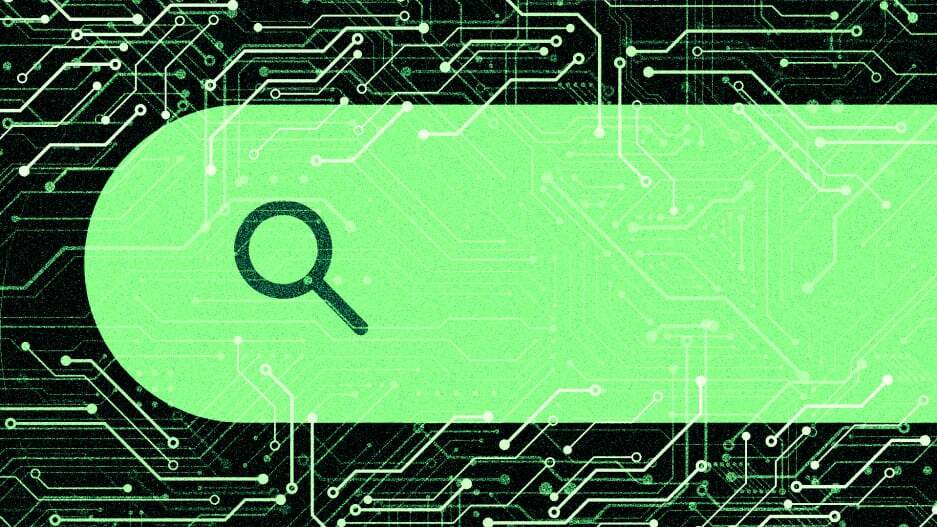
Google’s stock price lost 3% of its value earlier this week after the New York Times published a story suggesting the search giant’s business might be threatened by generative AI. A group of new AI-powered search startups have emerged, each hoping that as powerful large language models (LLMs) improve, AI chatbots will prove a better way of scouring the web’s content than Google’s crawlers and ranking system. The most pedigreed among them, perhaps, is Perplexity AI.
Led by CEO and cofounder Aravind Srinivas, the San Francisco-based startup boasts a who’s who of AI luminaries among its investors: People like neural network pioneer and Turing Award winner Yann LeCun (now chief scientist at Meta AI), Ashish Vaswani (lead inventor of the transformer models that power new AI chatbots, including OpenAI’s ChatGPT), Jeff Dean (who currently leads Google’s AI research), OpenAI founding member Andrej Karpathy, and early AI angel investors Elad Gil and Nat Friedman.
Perplexity, like other new AI search-bots, including Microsoft with its Bing Chat, hopes to one day carve out a slice of the massive web search market that Google has so thoroughly dominated for 30 years. Google made $162 billion last year from search advertising and licensing. Perplexity says it already has 2 million monthly active users.
AI search-bots represent a fundamentally different approach to retrieving content from the web. Google works by matching search queries with relevant sites that its web crawlers have found and indexed, then ranking those content sources based on their popularity and other factors. AI “answer engines” (as Perplexity terms them) leverage the language skill of large language models (LLMs) to understand queries, then assemble customized, conversational answers based on content from the millions of web pages to which the LLM was exposed during training.
Actually, Perplexity uses both approaches. “When you type in a question at Perplexity it’s going to look into a search index and pull up the relevant web pages—the top few web pages and the snippets,” says Srinivas, who worked at both Google’s DeepMind and Google Research, then at OpenAI before founding Perplexity. “Then it’s going to pass it into a chatbot LLM that’s going to look at all that and find out what’s actually useful to the query.”
Srinivas says Perplexity’s secret sauce may be the careful balance it’s struck between indexing/ranking and LLM-based search. “It’s very hard to identify the sweet spot between a canonical search engine that always gives you the links—and it’s pretty trustworthy, but not very productive in terms of directly getting what you want—and a hallucinatory ChatGPT that’s very easy and fun to use but you may not find the trust to actually believe what it says.”
Perplexity’s deals with LLMs’ habit of hallucination (i.e. making things up) by placing a strong emphasis on citations and references. The results from the initial query and all follow-up queries are always accompanied by such notations, and they can be clicked upon to ask follow-up questions or dig deeper into topics.
“We come from an academic background and we have this core principle that when you write a paper you are not allowed to say things that you cannot cite,” Srinivas explains. “That’s a core tenet of Perplexity . . . we basically told the LLM to never say anything it cannot back up.”
Perplexity has some very pragmatic reasons for emphasizing citations. Over years of use, people have built up trust in Google search results (even if they have to scroll past all the “sponsored results” to get to them). They’ve no such trust in a new and little-known AI “answer engine” like Perplexity. Srinivas says his company will have to earn trust, and bringing search results accompanied by receipts is a good start.
“We are giving the user the ability to verify what we say in case we get it wrong or in case they don’t trust us,” he says.
Right now, Perplexity is still very small: it had five full-time employees at the beginning of this year, and now has 11 full-time equivalent workers plus a few contractors on the payroll. Srinivas says he expects the company to grow to around 20 full-time equivalent employees by the end of the year. After raising an estimated $3 million in a seed round last October, the company recently closed a $25.6 million round led by New Enterprise Associates—a fairly modest amount among AI startups. The upside is that Perplexity will likely have ample time to work on its product without much pressure or distraction.
The company, which very recently released an iOS app, hopes to eventually find a good model for selling ads around its unique flavor of AI search, something nobody else in the space has achieved. While it plans to keep the existing service free, Perplexity hopes to begin making money by selling service tiers containing advanced features for users that establish an account and sign in. The company, which already has 100,000 signed-in users, has just released a feature allowing those users to save and share their search threads (their back-and-forths with the answer engine), and share them as permalinks with others. Account holders can also edit the sources that should be relied upon for a particular search, he says.
These new features add a Wikipedia-style social aspect to search, a direction toward which Perplexity hopes to push toward in the future. Srinivas wants users to be able to add additional context to common search threads, and correct information that may lean toward opinion or lack citation.
Srinivas and others at Perplexity have, naturally, been thinking and talking a lot about directions the platform might grow in the future, as LLMs mature and get better. The future they imagine has human users playing an important role.
“[Y]ou actually need to build a product and a platform where humans and AI coexist and can learn together,” Srinivas says. “That is, humans learn by asking questions with AI, and start trusting it more as it backs up what it says, and as AI gets help from the humans in terms of feedback when it goes wrong.”