- | 9:00 am
How transparency shapes the future of decision-making in AI
At the World Changing Ideas Summit, experts discussed the extensive implications of AI and the challenges in responsible deployment
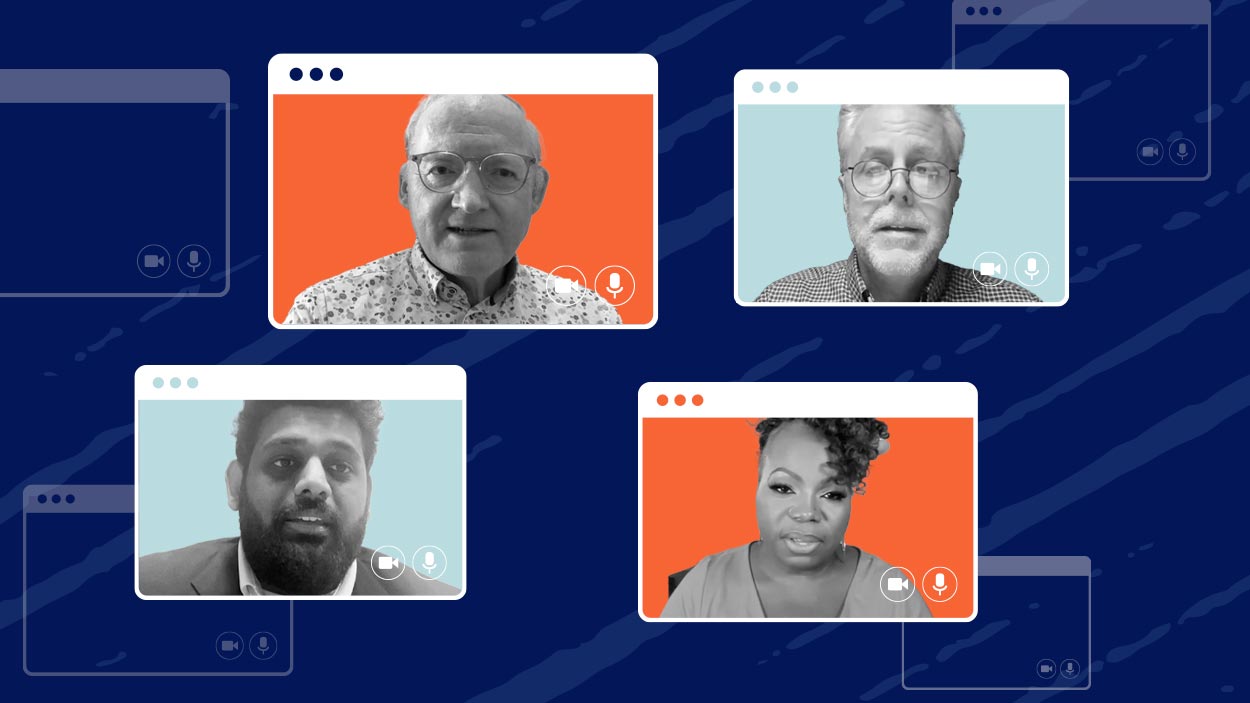
We keep reading about the impending technological revolution expected to reshape our lives, often overlooking that we are currently amid one of the most impactful transformations. Artificial intelligence has seen rapid development and will be much discussed in 2024.
But AI is much more than just crunched-in data. Adopting a meticulous approach to transparency and explainability in AI decision-making is imperative.
During the second edition of Fast Company Middle East’s World Changing Ideas Summit, global experts in AI shared their insights on the virtual panel, AI Ethics, Policies, & Regulations: How Can the Proper Guardrails Be Implemented?, elaborating on the extensive implications of AI and the practical challenges involved in the responsible deployment.
TRANSPARENCY AND EXPLAINABILITY
“We cannot ensure transparency for AI in one sense until all users can access and maintain some control over their data,” said John Havens, Author of Heartificial Intelligence & Hacking Happiness, Executive Director, The IEEE Global Initiative on Ethics of Autonomous & Intelligence Systems.
He talked about the importance of end-users having the ability to access and control their data for transparency in understanding the actions of these systems. According to him, embedding transparency and explainability into these systems primarily lies with the individuals developing the technology.
Expressing a contrarian view, Toby Walsh, Chief Scientist at UNSW AI Institute, argued that while desirable where achievable, transparency might be the most overrated ethical principle discussed in the context of AI systems. He said, “In certain contexts, transparency may indeed be undesirable. For instance, increased transparency might lead to manipulation in Google’s AI algorithms ranking search results. Additionally, there are settings where achieving transparency could be challenging or even impossible. Human vision, for instance, lacks full transparency in understanding its intricacies.”
However, Walsh suggested that this lack of complete understanding doesn’t preclude the ability to incorporate transparency into high-stakes systems. “By embedding these systems within trusted institutions, similar to human decision-making processes, we can ensure confidence in the outcomes they produce.”
Diya Wynn, Senior Practice Manager of Responsible AI at Amazon Web Services, said transparency is crucial to responsible AI. “This is evidenced by its inclusion in principle or strategy documents on responsible AI and trustworthy AI. Legislators are also emphasizing the importance of considering mandates on transparency as one of the guardrails for ensuring the safe and fair use of AI.”
But, whether AI is employed for decision support or decision making, it is crucial to integrate transparency and explainability from the beginning. This proactive approach is vital for establishing trust with users and organizations embracing AI. “Over the past several years, there has been a focus on approaches such as feature stores and feature engineering,” says Lyric Jain, CEO at Logically.
He believes noteworthy solutions are emerging even in supposedly “black box” approaches, such as large language models.
He added, “For instance, running a primary model for inference alongside a secondary model dedicated to transparency. This secondary model focuses on the inputs and outputs of the primary model, contributing to increased transparency even in the opaquest approaches.”
What is crucial is a conscious decision to invest in building and using AI in a transparent and explainable manner, especially in sensitive use cases.
CAN AI EVER HAVE EMPATHY?
Machines lack the inherent capacity for emotions or consciousness, said Havens. “Any belief that machines are or could be sentient is a matter of faith or informed speculation. As of today, attributing emotions to machines is termed anthropomorphization. In effective computing, this practice can lead to manipulation, particularly with the elderly or young people.”
This implies that attributing emotions to machines is more of a personal belief. Treating machines as if they have feelings, especially when dealing with the elderly or young people, can be misleading and potentially manipulative.
It’s crucial not to state that machines inherently possess ethics, emotions, or empathy. Instead, the emphasis should be on the humans creating the technology, who have empathy and compassion, and end users.
On the other hand, Walsh believes teaching machines ethics to embed the values we want in our systems is possible. “I am opposed to striving for empathy or compassion in machines. These qualities are distinctly human values and not inherent to machines. Machines lack sentience, consciousness, and emotions and can never genuinely exhibit empathy or compassion.”
This is a fundamental reason why we should refrain from entrusting high-stakes decisions that demand human empathy and compassion to machines. Such decisions are inherently human and should remain under the purview of humans.
For Jain, teams and individuals involved in building AI must be fully aware of how the technology they are developing will be utilized.
It is imperative to provide them with training in AI ethics, apprise them of the risks associated with AI, and instill a sense of responsibility in their AI development practices.
But, he believes that the effort doesn’t conclude there.
“We need to ensure that teams and individuals building AI are fully aware of how the technology they’re building will be used. We need them to be trained on AI ethics, the risks of AI, and responsible ways in which they need to go about building AI. It doesn’t stop there. We also need to ensure that users interacting and adopting AI have a baseline literacy level in interacting and dealing with such technology. So, looking at every human interaction with AI, from build to deployment to training to usage, we need to ensure a greater level of literacy and understanding of risks and AI ethics.”
In the end, Wynn leaves us with a question. “If machines mimic emotional qualities or compassion, does that mean they emote or have empathy without debating the philosophical?”
The question we want to ask: Why do we want machines to have ethics, empathy, or compassion? And we want that because we want to be able to eliminate or reduce the possibility of harmful effects or negative or unintended impacts of these systems on human beings.
“And for our society, if that’s the question, it’s true that we can program or design machines to adhere to ethical guidelines. We can design, train, implement, and use them in ways that prioritize safety, security, fairness, and inclusivity. I believe this is the objective of responsible AI,” said Wynn.
THE HUMAN TOUCH IN ALGORITHMS
Yes, but there are limitations, believes Jain. Context can be integrated through human and expert knowledge, embedded into discrete tasks that AI will perform or a series of tasks within a broader scope. He adds that it can be incorporated by ensuring there’s an element of human or expert-in-the-loop compatibility.
However, Havens argued algorithms cannot take human context into account. Human designers must consider human context and create algorithms incorporating these aspects.
“But again, I’m working hard to not separate what are essentially mathematical formulas, beautiful mathematical formulas, and say that they are human.”
ENSURING ETHICAL AI
Wynn said whether a dataset is fair and balanced depends on the application context and its use case. A crucial step in this process is defining what is considered fair from the beginning of the initial stages of design.
“ Responsible AI should be intentional and addressed in the early stages or at the inception of a system or product design. Discussing fairness involves eliminating or reducing bias in the system’s outcomes and ensuring consistent performance.”
This involves addressing statistically insignificant variations in results across different subgroups or populations. However, they might be defined. “ Biases can manifest in various aspects and are not exclusively limited to data. Biases can exist in how the data is labeled, the groups of people annotating it, the algorithms, and how we evaluate them. Therefore, data sets must accurately mirror the real-world environment in which the model is utilized.”
On the other hand, Jain said we must begin by ensuring a good understanding of where AI will be employed. Then, ensuring that the datasets used to train AI represent the situations where AI will be deployed and utilized is crucial. “While achieving complete representation may not always be possible, diligent efforts should be made, especially for high-impact scenarios. This includes ensuring extensive coverage of weighted scenarios that could lead to harm or potential abuse. Regarding abuse, it is essential to be mindful of areas such as disinformation.”
Havens added that all technology and technology policies must prioritize ecological flourishing and human well-being. “This implies that, as a component of human well-being, we must acknowledge the inherent bias in the datasets we use.”