- | 3:00 pm
How AI can help predict weather in the era of climate change
As conditions become more chaotic, scientists are looking to AI to help with the forecast
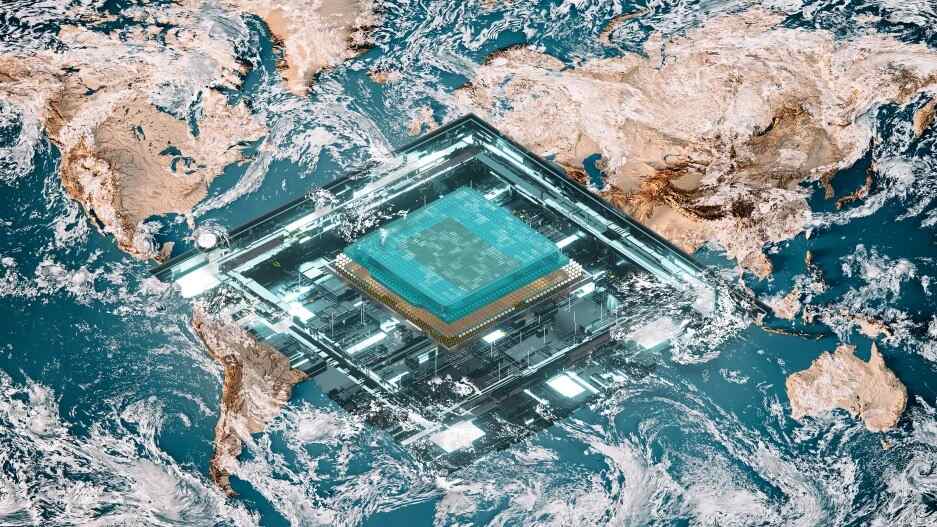
From floods in California to freezing temperatures in Texas, 2023 has already seen its share of unusual weather. As climate change intensifies, extreme weather events like heat waves, hurricanes, and droughts will continue to become more common.
As weather conditions change, it’s essential to know what’s coming. We need accurate weather forecasts on many different time scales in order to prepare for climate-related disasters, manage the power supply, plan agriculture, keep transportation running smoothly, and much more. However, we find ourselves in a catch-22, because as weather conditions change and intensify, they become even harder to forecast.
It isn’t all doom and gloom, however. Scientists are increasingly looking to AI to help us predict the weather, and some of the most promising approaches come from blending AI with existing scientific knowledge. Better forecasts play an important role in our efforts to combat climate change, a mission that is at the center of my work as a data scientist and co-founder of an initiative called Climate Change AI.
Our earliest attempts to predict the weather involved calculations based on the laws of physics using simple measurements of temperature and barometric pressure. Now forecasts are made by supercomputers processing vast amounts of data gathered from radar, satellites, weather balloons, ocean buoys, and surface-level sensors.
Weather prediction today is miles ahead of the past. The UK’s weather agency says our current four-day forecast is as accurate as the one-day forecast 30 years ago. But climate change makes it more difficult to predict the future based solely on past data and patterns, due to a statistical phenomenon known as distribution shift. A 2021 study found that warming of just a few degrees Celsius could shave about a day off our window of reliable forecasting.
This is where AI comes in. It shines at identifying patterns in massive amounts of data and predicting what will come next. AI excels at “nowcasting,” which is weather-speak for what’s coming in the next few hours. For example, the AI company DeepMind built a deep-learning tool that beat out existing physics-based models at predicting rain in the next hour and a half.
As we look beyond what’s going to happen this afternoon, both traditional and AI-driven models become less reliable. This is especially true for what are known as subseasonal forecasts that look around 15 to 45 days out. We’re good at predicting the weather tomorrow, and existing physics-based models do a decent job with longer-term forecasts that look at overall trends, say, a year from now. But forecasts in the medium term are trickier, especially in the face of changing weather patterns.
Some of the most promising approaches for subseasonal forecasting combine the best of both worlds by blending physics- and AI-based models. Our team at Climate Change AI recently co-organized a datathon run by Stanford University’s Women in Data Science initiative on this exact problem. The annual WiDS Datathon brings together thousands of participants from nearly 100 countries. This year, participants are developing solutions that combine existing scientific knowledge with AI models to improve medium-term weather prediction.
One approach makes forecasting quicker and more efficient by plugging AI components into existing physics-based models, which usually require massive amounts of data and processing power. The formation of clouds, for example, is much more complex and harder to model than you might expect. AI can provide cheaper approximations for complicated and costly cloud models, which can then be embedded into existing climate and weather forecasting systems.
Another approach is to build AI forecasting models with limits and parameters based on what we know about how weather operates in the real world. I do something similar in my research designing algorithms to integrate clean energy into our power grid. While AI is a great tool for this task, there are physical and engineering constraints associated with how the power grid operates that need to be incorporated into any AI-based model. In my work, this means structuring neural networks to ensure that their outputs meet certain physical criteria, in addition to the other factors for which they are optimizing.
Clean energy is one of many areas where accurate weather prediction, especially subseasonal forecasting, is crucial. A key obstacle to incorporating renewable energy into our power grid is that it is seen as less reliable than traditional energy sources like coal and natural gas, with solar and wind output at the whims of the day’s weather. But more accurate forecasting will allow us to better predict both energy supply (how much we can produce) and demand (how much we will use). This enables renewable sources to reliably meet our energy needs.
Better forecasts also help governments and communities anticipate and prepare for extreme weather events. Farmers can adapt planting and harvesting practices to avoid increasingly unexpected floods, droughts, frosts, and heat waves. Shipping and airline companies can plan around weather to ensure safe transport, and businesses can manage weather-related disruptions in their supply chains. And all of us can better plan trips, activities, and gatherings that depend on the weather.
Some of the flashiest gains in AI have taken place in areas where we have massive amounts of data. Natural language processing models like ChatGPT were trained using text drawn from the Internet, and computer vision tools like DALL-E were similarly trained with images. As we start to use AI for a broader set of societal challenges, we need to be cognizant of the expense, complication, and ethics of acquiring similarly large amounts of data in other arenas. We can reduce the amount of data we need by finding creative ways to combine the power of AI with our wealth of existing scientific knowledge.
This combination of innovative technology and human wisdom is the best way to harness AI to help us tackle the challenges of the future, especially climate change. It will be crucial as we learn to live with a changing climate and make the unexpected just a little more predictable.