- | 9:00 am
Generative AI’s gut check moment
Large language models still come with a number of vexing flaws that hinder their application in the real world.
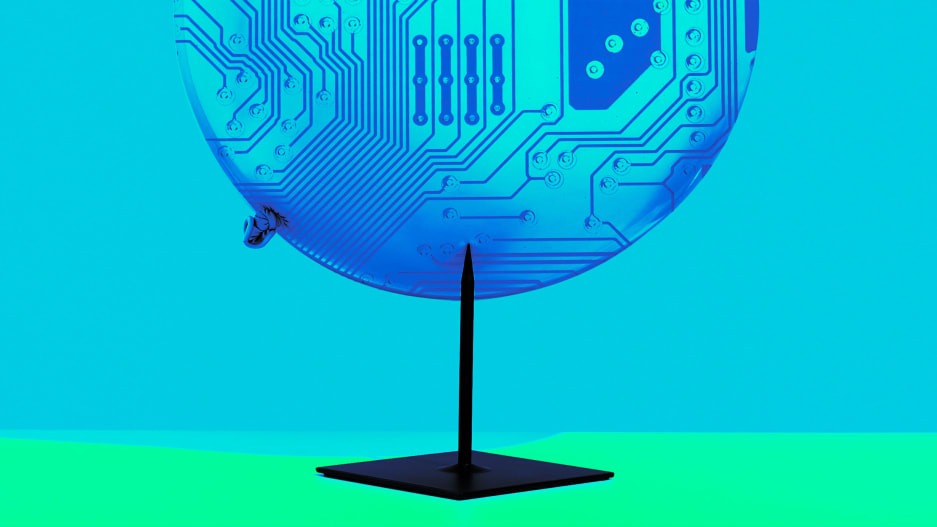
Welcome to AI Decoded, Fast Company’s weekly LinkedIn newsletter that breaks down the most important news in the world of AI. I’m Mark Sullivan, a senior writer at Fast Company, covering emerging tech, AI, and tech policy.
This week, I’m taking a step back to look at the massive hype around generative AI, and discussing why we may want to temper our expectations for the tech—at least in the short term.
SWIMMING AGAINST THE GENERATIVE AI WAVE
The arrival of ChatGPT in November 2022 set off an explosion of enthusiasm that large language models might reinvent the way businesses perform key functions, and in doing so would change the role of human workers. And indeed it’s been clear from the start that LLM-powered tools like ChatGPT, Bard, and Claude could help us compose emails, summarize documents, and even brainstorm ideas.
But, critics were quick to point out, LLMs also invented facts as impressively as a gifted teenager with a flair for BS, which seemed to make them unfit for critical tasks. That makes the tech in some ways unreliable, and would seem to mean it would need to be closely supervised in a business setting.
Seven months later, LLMs still face a hurdle with so-called “hallucinations,” along with a host of other issues, and progress toward solutions has to date been slow. Part of the problem, as I wrote in May, is that nobody knows exactly how LLMs work: At a base level, they are essentially auto-complete tools that guess the most likely next word in a sequence, but the inference process they go through to find the next words is extremely complex—so complex that not even the LLMs’ creators can explain what’s happening.
There is debate about whether or not a single LLM can be at once creative and completely trustworthy. The mechanism that lets LLMs improvise and hallucinate is the same one that allows them to make things up.
While many assume that LLMs are the path to Artificial General Intelligence—a hypothetical stage wherein the AI system can accomplish the same intellectual tasks as humans—some doubt that today’s biggest language models can truly reason through problems. Other studies call into question whether LLMs can ever be cleansed of bias.
Yet despite any shortcomings, the generative AI hype continues. The McKinsey Global Institute believes generative AI will create $2.6 trillion to $4.4 trillion of wealth in the global economy. The hype helps prop up the valuations of generative AI startups; and VCs use it as a basis for investing, sometimes in lieu of a solid business case from the startup. Hopefully, generative AI’s glow will extend to other types of AI companies that use smaller, more specialized models to tackle problems.
OPENAI LAUNCHING A WEB CRAWLER TO FETCH REAL-TIME INFO FROM THE WEB
The LLM behind ChatGPT was trained on massive amounts of content scraped from the internet (without permission from publishers)—a process that was made possible via either a third-party web crawler or a homegrown, nonpublic tool. Now, ChatGPT-maker OpenAI has announced its own web crawler, GPTBot, that could allow for web content collection in a more accurate and safer fashion.
GPTBot is likely an attempt by OpenAI to be more transparent about the means by which it gathers training data, and perhaps to atone for its past sins. The company now faces at least two lawsuits over its data scraping practices, and it last month agreed to a licensing deal with the Associated Press to use its content for training. In the GPTBot release notes, OpenAI provides explicit instructions for publishers who want to block the bot from accessing their sites—an attempt, perhaps, to appease regulators who are taking a keen interest in the rights of content owners in the AI age. In addition, OpenAI says the crawler will avoid paywalled sites, and sites that contain personally identifiable information or content that violates its usage policies.
FALLOUT AT STABILITY AI AFTER SCATHING FORBES PIECE
In early June, Forbes published an article about Emad Mostaque, the charismatic leader of Stability AI, the U.K.-based company behind last year’s breakout AI image generator Stable Diffusion. After talking to 30 people surrounding Stability and its leader, Forbes reported that Mostaque had raised hype and funding for his company in part by stretching the truth, both about the real accomplishments of the company and about his own background.
Bloomberg’s Mark Bergen and Rachel Metz revisited the topic this week, talking to a large group of current and former Stability employees as well as investors, vendors, and contractors. The sources described a “disorganized company helmed by an inexperienced CEO with a history of outlandish claims and lofty promises that don’t always come to fruition,” as Bergen and Metz wrote. Because of that, Stability AI lost some critical researchers and executives—just when it badly needed to scale the company and attract new investment money. It raised a $101 million seed round at a $1 billion valuation last year. The company has been trying to raise more money this year at a $4 billion valuation, Bloomberg reports, but has been unsuccessful so far.
Stability AI has denied or dodge every claim made in the Forbes and Bloomberg pieces. But it remains that the initial tailwinds it enjoyed after the release of Stable Diffusion have vanished, and the company is struggling to stay on the front of the current generative AI wave.